School Administrator Magazine
AASA's award-winning magazine provides big-picture perspectives on a broad range of issues in school system leadership and resources to support the effective operation of schools nationwide.
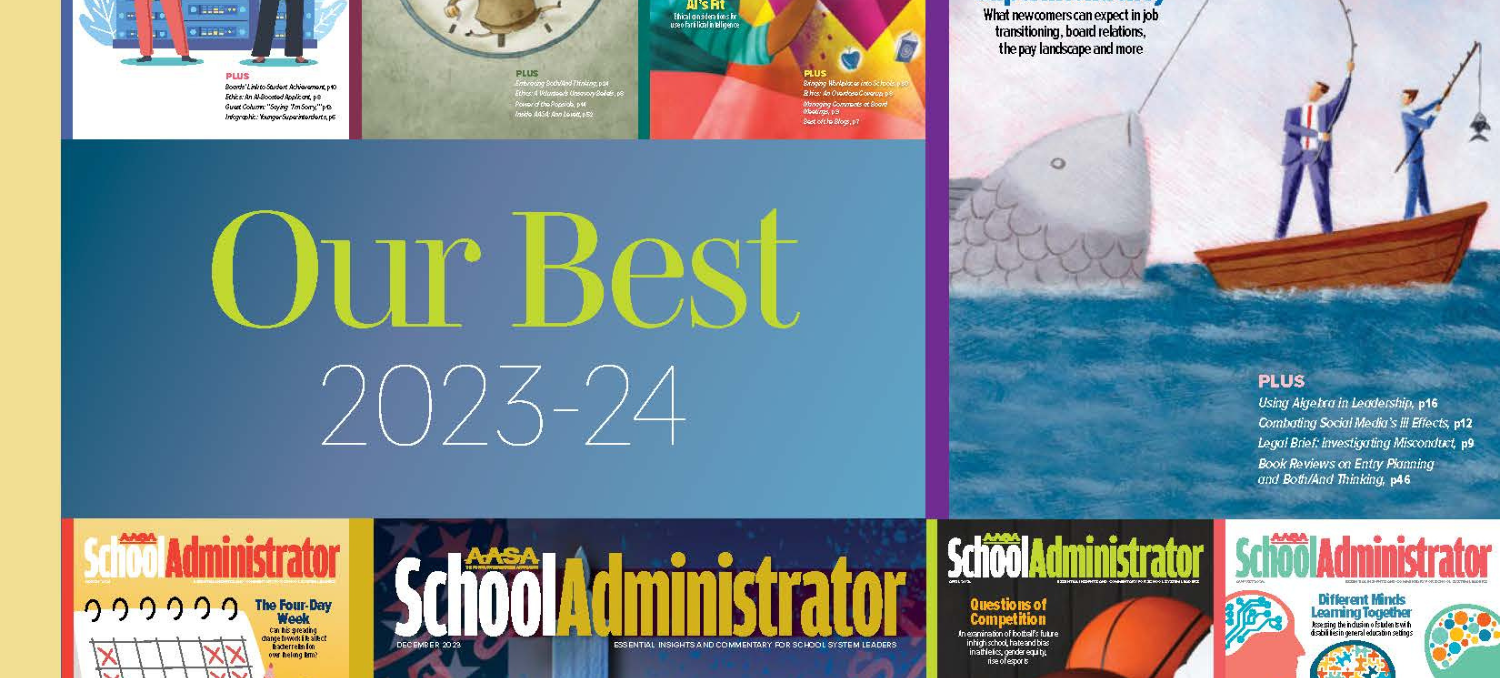
Current Issue
School Administrator: Best of 2023-24
Advertisement
Previous Issues
-
July 2024: School Administrator
This digital-only issue compiles articles and columns of the past year examining changing mindsets, superintendent mental health and the importance of data privacy and cybersecurity and much more.
-
June 2024: School Administrator
This issue examines how school districts can modify attitudes about cellphones in schools, staff training and inclusion of all children with disabilities to improve teaching and learning experiences.
-
May 2024: School Administrator
This issue examines several solutions and strategies to help school districts hire and retain teachers amid ongoing staff shortages.
-
April 2024: School Administrator
This issue examines how school districts can modify behavioral and operational practices to account for climate change.
-
March 2024: School Administrator
This issue examines the rise of the four-day school week and the digital learning landscape in the aftermath of COVID-19.
-
February 2024: School Administrator
This issue examines how superintendents are defending their school districts from cyberattacks and protecting their students’ data.
-
January 2024: School Administrator
This issue examines some distinctive ways that public schools deliver health services to their communities today.
-
December 2023: School Administrator
This issue examines the role superintendents can play in legislative advocacy on state and federal levels.
School Administrator Staff
Advertise in School Administrator
For information on advertising with AASA, contact Kathy Sveen at 312-673-5635 or ksveen@smithbucklin.com.
Advertisement
Advertisement